AiCAMstir Kick-off Meeting, 29 July 2021: Difference between revisions
Jump to navigation
Jump to search
No edit summary |
No edit summary |
||
Line 168: | Line 168: | ||
[[File:AiCAMstir Kick-off Meeting, 29th July 2021 - Slide 18.JPG|thumb|left|upright=3|alt=|Example: | [[File:AiCAMstir Kick-off Meeting, 29th July 2021 - Slide 18.JPG|thumb|left|upright=3.2|alt=|Example: | ||
β’ Thick plate Rosenthal gives: [[FSW_Power#Power.2C_.7F.27.22.60UNIQ-MathJax28-QINU.60.22.27.7F |Power π]] | β’ Thick plate Rosenthal gives: [[FSW_Power#Power.2C_.7F.27.22.60UNIQ-MathJax28-QINU.60.22.27.7F |Power π]] | ||
]] | ]] |
Revision as of 15:08, 30 July 2021
The aiCAMstir Kick-off Meeting was held online on 29th July 2021 with 16 attendees.
List of Attendees (alphabetically sorted by first name)
Company | People | Status | Signed-up on |
---|---|---|---|
Please enter your company name | Azman Ismail - Ts. Dr. | Guest | |
Please enter your company name | Breno Boretti Galizoni | Guest | |
Please enter your company name | Erhard Buchmann | Guest | |
Please enter your company name | Gokhan Tekin_Alcomet_R&D_Director (Konuk) | Guest | |
LAMEF | Guilherme V. B. Lemos | R&D Institute | 2021-06-01 |
Latrock GmbH | Heorhii Vdovychenko | Data science, machine learning etc | 2021-07-12 |
Sabe Technology Ltd | Josselin Guillozet | Consultancy service provider | 2021-02-18 |
Please enter your institute's name | Koen Faes | R&D Institute | 2021-04-14 |
Please enter your company name | Laurie Da Silva | Guest | |
FTS Engineering Answers Ltd | Mike Lewis | Consultancy service provider | 2021-01-14 |
Please enter your company name | Mansoor, Bilal | Guest | |
Please enter your company name | MJ Sefat | Guest | |
TPO Maharashtra Institute of Technology Aurangabad | Please enter your name | Guest | |
Please enter your institute's name | Renan Landell | R&D Institute | 2021-06-07 |
Please enter your institute's name | Sandeep | R&D Institute | 2021-07-25 |
Please enter your company name | Shivraman | Guest | |
AluStir | Stephan Kallee | Consultancy service provider | 2021-01-14 |
Agenda of the aiCAMstir Kick-off Meeting: β’ Welcome and introduction (2-3 sentences each)
β’ Computer Aided Manufacturing of friction stir welds - The vision (Stephan Kallee, AluStir)
β’ Computional Fluid Dynamics (Mike Lewis, FTS Engineering Answers Ltd)
β’ Artificial Intelligence and machine learning algorithms (Josselin Guillozet, Sabe Technology Ltd)
β’ Analytical models (Simon Smith, Transforming Stress Ltd)
β’ Friction Stir Welding (LAMEF/UFRGS)
β’ Two companies β one team (Smart Industry Group and Latrock GmbH)
β’ Needs and contributions of the attendees (3-5 sentences)
β’ Discussion
β’ Organisational comments and date of next meeting
β’ Computer Aided Manufacturing of friction stir welds - The vision (Stephan Kallee, AluStir)
β’ Computional Fluid Dynamics (Mike Lewis, FTS Engineering Answers Ltd)
β’ Artificial Intelligence and machine learning algorithms (Josselin Guillozet, Sabe Technology Ltd)
β’ Analytical models (Simon Smith, Transforming Stress Ltd)
β’ Friction Stir Welding (LAMEF/UFRGS)
β’ Two companies β one team (Smart Industry Group and Latrock GmbH)
β’ Needs and contributions of the attendees (3-5 sentences)
β’ Discussion
β’ Organisational comments and date of next meeting
Avoid:
β’ Wrong parameters
β’ Simple tools
β’ Inappropriate machines
β’ Chamfer, radius or taper on workpieces
β’ Insufficient clamping
Reasons for low-quality welds:
β’ Downward pressure is too low
β’ Welding speed is too fast or slow
β’ Rotation speed is too high or low
β’ Tool position is too high
β’ Tool rotates in the wrong direction
β’ Gap between the work pieces
β’ Wrong parameters
β’ Simple tools
β’ Inappropriate machines
β’ Chamfer, radius or taper on workpieces
β’ Insufficient clamping
Reasons for low-quality welds:
β’ Downward pressure is too low
β’ Welding speed is too fast or slow
β’ Rotation speed is too high or low
β’ Tool position is too high
β’ Tool rotates in the wrong direction
β’ Gap between the work pieces
FSW Simulation using Computerised Fluid Dynamics (CFD) β Butt Weld
Torque Comparison:
β’ Test = 38 Nm
β’ CFD = 30 Nm
Heat Input Comparison:
β’ Test = 2000 W
β’ CFD = 1800 W
FTS is also involved in: β’ Standard Setting (energy Institute Subsea Guidelines)
β’ Joint Industry Projects
:β’ Multiphase FIV JIP
:β’ Multiphase FIV SIG
β’ Many trouble shooting projects
Torque Comparison:
β’ Test = 38 Nm
β’ CFD = 30 Nm
Heat Input Comparison:
β’ Test = 2000 W
β’ CFD = 1800 W
FTS is also involved in: β’ Standard Setting (energy Institute Subsea Guidelines)
β’ Joint Industry Projects
:β’ Multiphase FIV JIP
:β’ Multiphase FIV SIG
β’ Many trouble shooting projects
FSW Simulation using Computerised Fluid Dynamics (CFD) β Butt Weld
Comparison with Aldanonda Work for conventional thread at 1200 rpm and 250 mm/min www.mdpi.com/2075-4701/10/7/872
Comparison with Aldanonda Work for conventional thread at 1200 rpm and 250 mm/min www.mdpi.com/2075-4701/10/7/872
β’The first step is the construction of a high-quality dataset of good and bad welding. This dataset may consist of experimental and/or numerical samples.
β’Machine learning models could then be trained to predict the right set of tool parameters to achieve a good welding (supervised learning)
β’ Input parameters (features) of the models may be:
::β’ Physical quantities and derivatives
::β’ Time series of these same physical quantities
::β’ Images/videos
β’ Output (target) may be a continuous variable like the torque (regression) or whether the welding is expected to be good or bad (classification)
β’Machine learning models could then be trained to predict the right set of tool parameters to achieve a good welding (supervised learning)
β’ Input parameters (features) of the models may be:
::β’ Physical quantities and derivatives
::β’ Time series of these same physical quantities
::β’ Images/videos
β’ Output (target) may be a continuous variable like the torque (regression) or whether the welding is expected to be good or bad (classification)
β’ βNon-deepβ learning models like Linear Regression, Support Vector Machine or Decision Trees may be directly used with well-designed input features. The model may be inspired by equations of the expected physics.
β’ Signal/Image processing and Computer Vision methods may help extract relevant features from images or videos.
β’ Deep Neural Networks may be used to extract more subtle patterns from images/videos or time series (e.g. Convolutional Neural Network - CNN).
β’ Signal/Image processing and Computer Vision methods may help extract relevant features from images or videos.
β’ Deep Neural Networks may be used to extract more subtle patterns from images/videos or time series (e.g. Convolutional Neural Network - CNN).
Baseline variables for analysis
β’ Temperature, π is a function of the unknown power, and:
::β’ Distance from tool axis, π₯
::β’ Travel speed, π£
::β’ Thermal properties, conductivity, π and diffusivity, π
::β’ Room temperature, π0
β’ Strength, π is a function of the unknown temperature, and:
::β’ Room temperature strength, ππ π
::β’ Material melting temperature, ππ
β’ Torque, π‘ is a function of the unknown material strength near the tool, and:
::β’ The radius of the tool, π
β’ FSW power is equal to the unknown torque, π‘ times the rotation speed, ω
β’ Temperature, π is a function of the unknown power, and:
::β’ Distance from tool axis, π₯
::β’ Travel speed, π£
::β’ Thermal properties, conductivity, π and diffusivity, π
::β’ Room temperature, π0
β’ Strength, π is a function of the unknown temperature, and:
::β’ Room temperature strength, ππ π
::β’ Material melting temperature, ππ
β’ Torque, π‘ is a function of the unknown material strength near the tool, and:
::β’ The radius of the tool, π
β’ FSW power is equal to the unknown torque, π‘ times the rotation speed, ω
Assumed relationships:
β’ Temperature, π predicted using a Rosenthal equation, assuming power, π
β’ Strength, π assumed to be a known function of π between the room
temperature value, ππ π and zero at the melting point, ππ
β’ Torque based upon the need to βyieldβ the material with strength, π
β’ Power straightforward function of torque, π‘ and rotation speed, ω
β’ Hence:
::β’ Four equations
::β’ Four unknowns (π, π‘, π, π)
β’ Answer: FSW power based upon (π£, ππ π, ππ, π , ω, π, π)
β’ Temperature, π predicted using a Rosenthal equation, assuming power, π
β’ Strength, π assumed to be a known function of π between the room
temperature value, ππ π and zero at the melting point, ππ
β’ Torque based upon the need to βyieldβ the material with strength, π
β’ Power straightforward function of torque, π‘ and rotation speed, ω
β’ Hence:
::β’ Four equations
::β’ Four unknowns (π, π‘, π, π)
β’ Answer: FSW power based upon (π£, ππ π, ππ, π , ω, π, π)
Example: β’ Thick plate Rosenthal gives: Power π
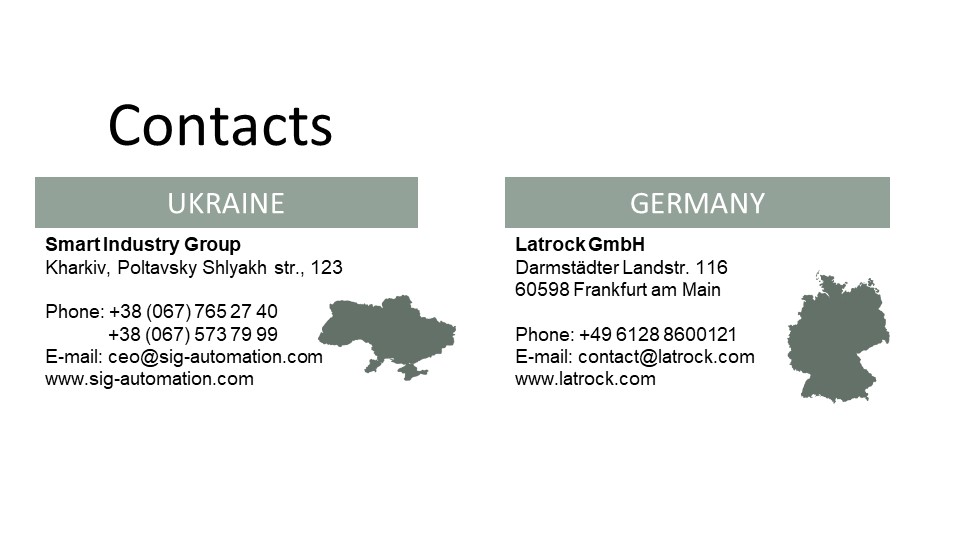
Contacts:
β’ Smart Industry Group, Kharkiv, Poltavsky Shlyakh str., 123, Phone: +38 (067) 765 27 40 and +38 (067) 573 79 99, E-mail: ceo@sig-automation.com, www.sig-automation.com
β’ Latrock GmbH, DarmstΓ€dter Landstr. 116, 60598 Frankfurt am Main, Phone: +49 6128 8600121, E-mail: contact@latrock.com www.latrock.com
β’ Smart Industry Group, Kharkiv, Poltavsky Shlyakh str., 123, Phone: +38 (067) 765 27 40 and +38 (067) 573 79 99, E-mail: ceo@sig-automation.com, www.sig-automation.com
β’ Latrock GmbH, DarmstΓ€dter Landstr. 116, 60598 Frankfurt am Main, Phone: +49 6128 8600121, E-mail: contact@latrock.com www.latrock.com